Summary:
In 2021, researchers at MIT and McKinsey teamed up to ask more than 100 companies how they were using AI in their operations and to learn what separated the highest-performing companies from the rest. They conducted a similar survey in 2023 to see what had changed. They found that the gap between leading companies and the rest had widened; that the payback-period for AI investments was much shorter; and that leading companies were better at identifying and implementing use cases that delivered positive outcomes with lower risk.
Three years ago, when we asked companies how they were using artificial intelligence in their operations, the highest-performing companies (the leaders), stood out in five areas: governance, deployment, partnerships, people, and data availability. Since then, generative AI (gen AI) has burst on the scene. So, in late 2023, we followed up, surveying more than 100 companies in sectors from automotive to mining and conducting in-depth interviews with senior executives.
We saw three notable developments. First, the gap between the leaders and the rest has widened. The leaders — defined as the top 25% of respondents — now see performance levels 3.8x that of the bottom half of companies, up from 2.7x in 2021. One reason for this is that as leaders build differentiated capabilities, these have a compounding effect over time, widening the performance advantage.
Second, the payback period for AI-related investments is much shorter — converging at just six to 12 months for all of the companies we surveyed. In 2021, only the leaders saw an impact in that timeframe, while the laggards took much longer, typically 18 to 24 months. Experience has been a successful teacher. Better governance practices have enabled companies to identify and implement the most promising use cases. Companies also now have more complete, more accessible, and higher-quality data, as well as a larger ecosystem of AI software solution providers. These can provide consistent results for a monthly fee, freeing companies from the need to make costly upfront investments. And as the value-creation potential of AI has become clear, there are fewer implementation and organizational barriers that in the past drove up costs.
Third, while there has been a tsunami of AI initiatives, the winners are better at identifying and implementing use cases that deliver positive outcomes with lower risk.
Our survey results suggested four factors underlying these developments:
1. Executive sponsorship
In our 2023 survey, more than three-quarters of AI leaders had C-level sponsorship — most of the time coming from the CEO or board. Determining the return on investment (ROI) for AI initiatives is difficult: The savings may not be immediate, and some of the benefits, such as freeing up employee time to perform more value-added work, are indirect.
It takes leadership to decide to move forward without clear expectations, while also directing resources to projects with the highest potential. For example, industrial manufacturer Cooper Standard’s initial machine-learning initiative fell short when a partnership didn’t click. But a senior-level champion stepped in to lead the in-house research that developed an AI-driven advanced process control manufacturing operation that worked so well it became a successful subsidiary business.
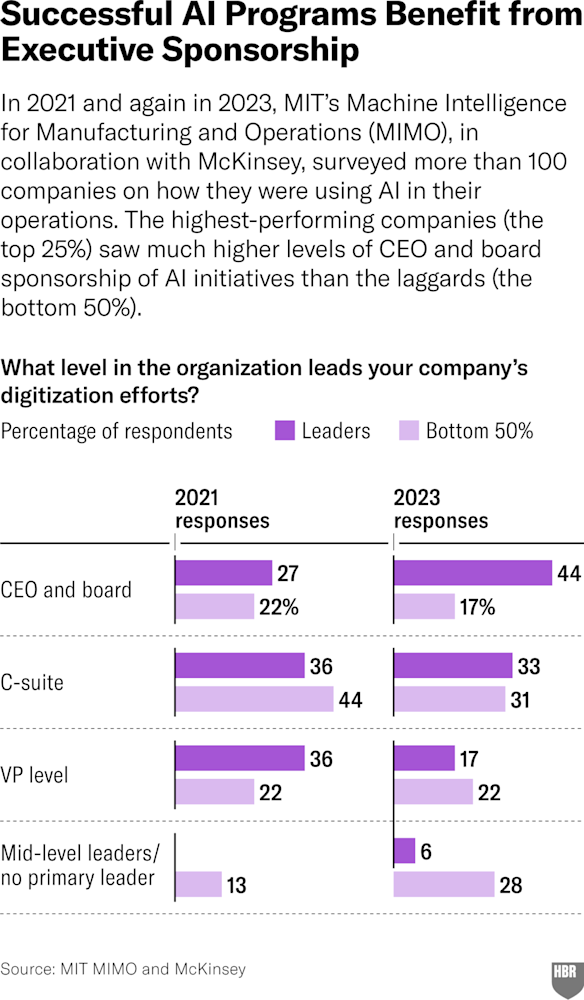
2. A network of partners
In-house capabilities are necessary; almost 90% of leaders in the 2023 survey reported using internal resources to develop AI solutions. But they’re often not sufficient: Two-thirds of leaders also had outside partners, typically to fill gaps in expertise and to accelerate development and payback.
What surprised us is that the kind of partnerships changed significantly. In our 2021 survey, academia and startups were the most common partners; two years later, respondents named a maturing ecosystem of consultants, vendors, and industry partners. The implication is that AI has matured enough that practical approaches are valued the most.
In addition, the extent and depth of cross-industry collaboration is notable, with leaders engaging with others through conferences, journals, and face-to-face meetings, allowing them to apply analogous use cases to their own operations. Before investing big in AI, for example, mining company Freeport-McMoRan visited companies in other industries, applying what it learned about how pharmaceutical companies use AI to map out molecules to its own work mapping chemical compounds.
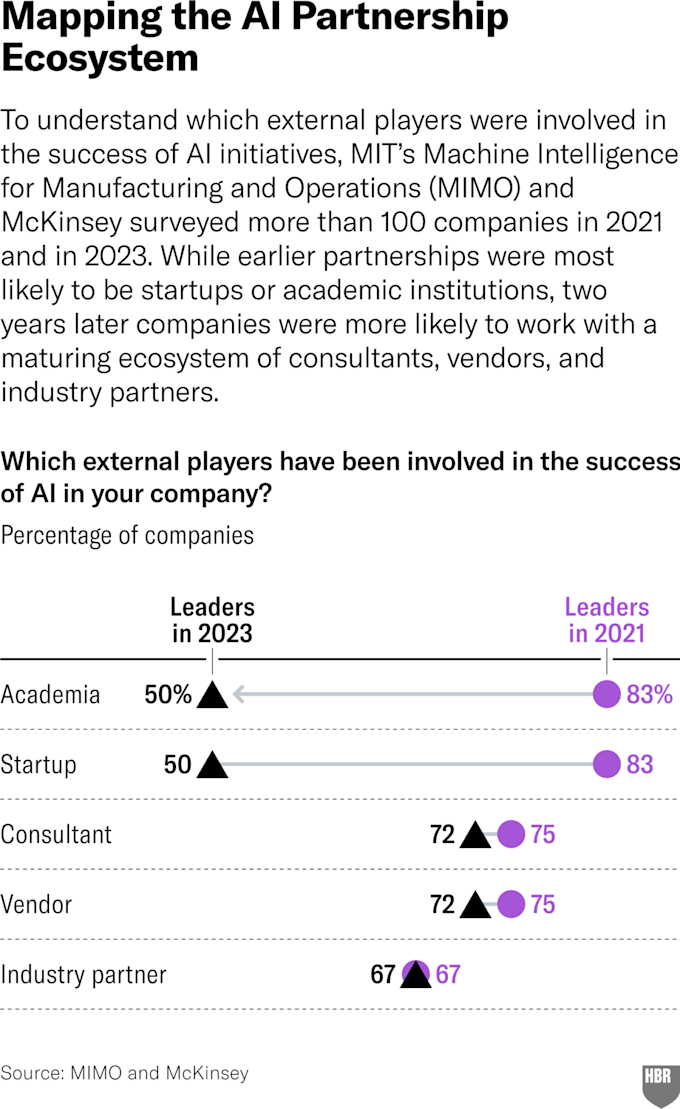
3. Cross-department communication
In successful companies, we saw smooth collaboration between IT and operations. One common approach is to create a “center of excellence” (CoE), a cross-silo internal organization staffed with employees with data-science skills. The CoE ensures that AI projects get implemented efficiently and deliver value, while also addressing issues such as cybersecurity, data error, and compliance. CoEs typically create standardized processes and keep the talent pipeline filled, through both hiring and capability-building. They can leverage the greater collaboration, talent, and diversity in experience that comes with a larger team. Almost six in 10 leaders surveyed have a CoE reporting at either the corporate or business-unit level.
In one implementation, a CoE at Target, a top 10 retailer by global revenue, took only six months to develop an in-store gen AI chatbot. A few months later, it was rolled out to their nearly 2,000 retail locations. The chatbot makes thousands of pages of best practices and manuals easily accessible to store employees, reducing training time, the demand for internal experts, and, perhaps, attrition.
An alternative approach is to create dedicated cross-functional teams of data scientists, operational gurus, and engineering expert within a business unit. Sometimes co-located at a production site, this group is given a mandate to address high-priority topics for the business unit, but with lower standardization with other parts of the company.
4. Data management
For companies to use AI well, they need accurate, pertinent, and well-organized data. Too often, though, they either don’t collect the right information, or it is so inaccurate and poorly managed that it isn’t useful.
The leaders we talked to invest in systems and processes to make sure that doesn’t happen. For a start, they are much more likely to record relevant equipment data, such as critical-to-quality process measurements, production rate, and downtime. Then they manage it meticulously, often in the cloud, allowing them to make informed decisions that drive efficiency and innovation.
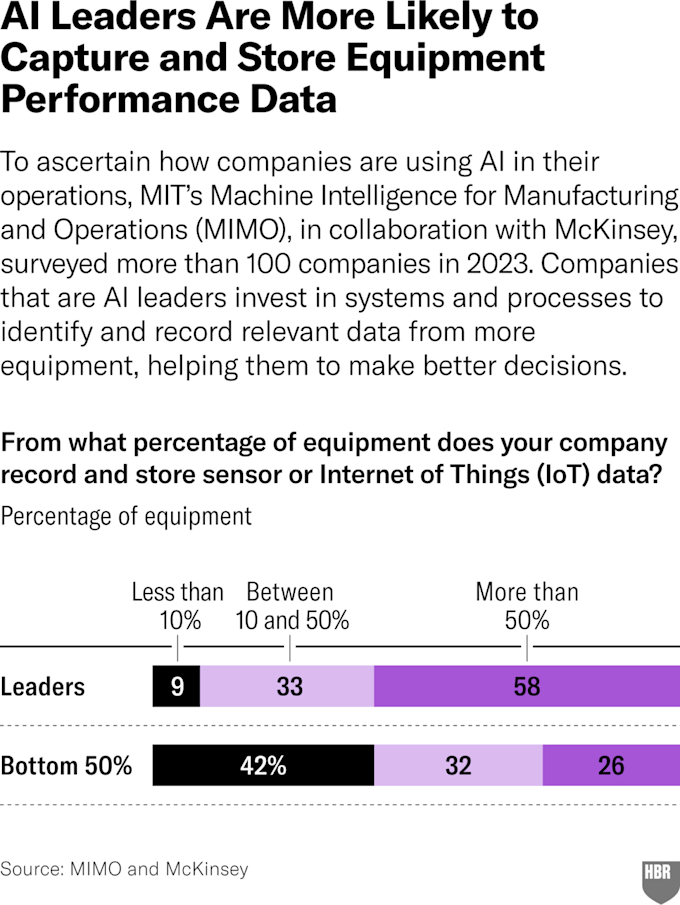
The results of such efforts can be substantial. To manage its data, Brussels-based Titan Cement integrated thousands of sensors, invested in cloud-based data storage, connected all its plants, and established a centralized data-science team, with sophisticated cybersecurity keeping everything secure. By tracking energy usage and production throughput, Titan improved both significantly. And by using its well-organized data to develop unique algorithms, it optimized production, improved reliability and streamlined logistics. All told, these efforts led to an estimated ROI of 500% for Titan.
With the rise of gen AI, unstructured text-based data can now drive rapid improvement and help fill the skills gap that many companies experience. For example, Panasonic Energy North America has used Palantir’s Artificial Intelligence Platform to create a maintenance assistant trained on 1 million tickets to assist 350 maintenance technicians in making 5.5 million batteries per day. The assistant delivers precise repair recommendations to front-line technicians by integrating insights from ticket history, maintenance manuals, machine telemetry, and captured expert knowledge. Technicians contribute to and refine the knowledge base in real-time, resulting in reduced machine downtime, greater throughput, and rapid onboarding of new technicians.
. . .
Capturing value from AI in operations is a challenge, and gen AI adds more complexity. But the rewards are real: McKinsey research has found that, across sectors, AI leaders deliver higher shareholder returns.
With more mature solutions and analytics tools, AI is increasingly accessible. Even digital and data science novices can now use machine learning tools, lowering the barriers to entry. Catching up, then, is a distinct possibility. To do so, the laggards would do well to focus on these four elements — essentially, to follow the leaders. If they don’t, they risk falling out of the competition altogether.
The authors would like to thank the following people for their contributions. MIT Leaders for Global Operations: Professors Duane S. Boning and Retsef Levi, and Research Scientist Ben Armstrong. At McKinsey: Kyle Danner, Arnav Dey, Pete Kimball, and Delphine Zurkiya. MIT students: Caeley Harihara, Rachael Harkavy, Ryan Kochert, Dave Lauzun, Carla Lorente, Max Malinowski and Will McNulty.
Copyright 2025 Harvard Business School Publishing Corporation. Distributed by The New York Times Syndicate.
Topics
Technology Integration
Systems Awareness
Critical Appraisal Skills
Related
Social Loafing and the Healthcare Team4 Questions to Ask Before You Invest In a Workplace Wellness AppHow to Spot an Incompetent LeaderRecommended Reading
Operations and Policy
Social Loafing and the Healthcare Team
Operations and Policy
4 Questions to Ask Before You Invest In a Workplace Wellness App
Problem Solving
How to Spot an Incompetent Leader
Problem Solving
Connect Your Learning Programs to Your Company’s Strategy
Strategy and Innovation
Health Policy: What’s On the Table for the Second Trump Administration?
Strategy and Innovation
Mindbending Drugs: Psychedelics, Cannabis, and Physician Leadership