Abstract:
The operating room (OR) management literature tends to view management problems as having finite solutions and assumes that equilibrium exists in the intricate encounters that occur every day. In this article, we review complexity theory and assess its applicability to the strategic, tactical, and operational issues facing OR managers. By building on complexity theory and its assumptions, we also show that as complex systems, ORs resemble high-reliability organizations more than they resemble ultra-safe organizations. This distinction and the limitations of the current, linear modeling may have potential implications for the future of OR management research and practice. Opening the door to complexity, understanding the underpinnings of high-reliability organizations, and admitting that OR systems are complex adaptive systems will lead to self-governing, transparent processes that envision the OR as a living, growing, sustainable human endeavor.
Introduction: The Operating Suite as a Complex Adaptive System
All complex, adaptive systems—economies, minds, organisms—build models that allow them to anticipate the world. —John Holland
Organizations carrying on the most complex types of work develop formal systems that are designed to be resistant to change and to sustain the status quo or stability in the interest of efficiency. The well-defined hierarchical structures, the rules and procedures, and the machinery of repetitive, routine activities of most organizations are oriented toward stability and a more predictive way of functioning to achieve a somewhat seamless objective every day.(1) This leads to decision-making models that are intended to optimize efficient use of resources to improve organizational performance. Even with significant volatility in market environments and unpredictability in some of the organizational inputs, firms try to establish some level of equilibrium to support their decision-making. Many economic models presume equilibrium states even if the real world is dynamic.(2) The possibility of a finite set of solutions in such an equilibrium state often is implicit in the operating room (OR) management literature as it seeks to develop optimal solutions.(3,4) This presumed equilibrium is seen in the proscriptive management tools of block allocation and daily scheduling model.
However, almost all human organizations have varying levels of instability. Instability enters into organizations through different means, such as the influence of other organizations or partners, human resources, and patterns of behaviors and relationships. Although organizational behavior in response to instability may not be possible to predict in advance, over the long term organizations tend to develop uniformity or structure—known as bounded instability. Instability and bounded instability are, according to complexity theory, part of the fundamental nature of organizations that strive to operate with an innovative culture and be creative in developing systems. For example, Stacey(5) notes that “in order to produce creative, innovative, continually changeable behavior, systems must operate far from equilibrium where they are driven by negative and positive feedback from paradoxical states of stability and instability, predictability, and unpredictability.” Under these conditions, innovative organizations are expected to exhibit not planned but emergent change behavior. Such an emergent change is based on the assumption that change is continuous and open ended, an unpredictable process of aligning and realigning organizations to their changing environments.(6) In truth, this is merely a representation of complex adaptive systems (CASs) where a group of individual agents, each in communication, competition, and conditional interaction with others, helps lead an emergent behavior that is not a by-product of simply adding individual actions.(7) This article is a review of complexity theory (CT) as applied to the OR viewed from the “emergence” perspective.
In the natural world, systems such as brains, immune systems, cells, and ant colonies are complex adaptive systems. In the human world, cultural and social systems—such as political parties, scientific communities and economies—are similar.(8) Returning to the example of economics, the magnitude of complexity scales when considering local and regional systems versus national and international ones.(9) This is critical for individuals involved in the management of perioperative processes to understand because we believe that ORs are CASs. Now that we have defined CASs, let us apply the theory to the OR to see if it fits.
CASs are made of agents. In each of the system examples listed above, there are many “agents” exerting influence. In these networks, control is highly dispersed. In the OR environment, surgeons, anesthesia providers, and nurses all possess different hierarchies, and each constituency has different priorities and goals. An institutional surgical services committee may consist of representatives from each of these stakeholders.
CASs are multilevel. A CAS has many levels of organization. Agents at any one level serve as building blocks for agents at a higher level. These systems are constantly revising and rearranging their building blocks as they gain experience and systemic knowledge.
CASs prognosticate. An important aspect of CASs is that they anticipate the future. This is antithetical to most OR management studies. Countless studies have used historical data to make predictions about how to manage the OR in the future. Anticipating the future implies a very different skill set, similar to that used in managing a high-reliability organization (HRO).
CASs have specialization. CASs have many niches, each one of which can be exploited by an agent in order to fill that niche (e.g., DaVinci robotic systems, hybrid ORs). New opportunities are always being created by the system.
Viewed through this lens, ORs have all the components of a CAS. We believe that this framework must be taken into account in order to manage ORs effectively and efficiently.
Current Limitations of the Literature
Many studies in the OR management literature have applied calculus or linear analysis techniques.(10,11) Categorical variables—variables that can take on one of a limited, usually fixed, number of possible values—are used. These conventional mathematical modeling techniques are well suited to describing unchanging particles moving in fixed environments, static governmental budgets, and predictable production on manufacturing lines.
Over the past 50 years, however, it has been recognized that many, if not most, systems are more complex and require more sophisticated mathematical modeling and prediction tools than calculus or linear analysis typically provides. Analysis of CASs requires techniques that account for internal models, allow for the “emergence” of new building blocks or behavior patterns, and recognize the rich web of interactions between multiple agents (e.g., topography). Essentially, it is meaningless to discuss a CAS in equilibrium because the system can never get there. As Marion(12) says, “It is always unfolding, always in transition. In fact, if the system does reach equilibrium, it is dead.”
The reductionism in the current OR management literature limits applicability, because one size does not fit all. One classic example is the high-throughput delivery OR processes that benefit the surgeon.(13) In this case, careful patient selection and coordinated surgical workflows make the service line resemble a manufacturing process. However, this process is not necessarily transplantable and is unique to the specific characteristics of the local environment, institution, surgeon(s), and staff. In the real world, the management of a single OR is very different from the management of a perioperative process at a large academic institution.
In topographical terms, OR management has been viewed from the perspective of game theory. Some useful information from game theory (e.g., the “tragedy of the commons,” wherein an individual attempting to maximize access to a resource limits it to others, commonly seen in competition for surgical time and block allocations) has been gleaned, but the applications often are time-constrained, interaction-specific, and self-limiting. Many popular OR management trends have co-opted the management literature for production processes and applied them to the OR system. This one-to-one transplantation from one system to another may not be optimal. Even the Toyota Production System and LEAN management recognize the differences between manufacturing and service industries.(14) It has been insufficiently acknowledged that patients, staff, professionals, and institutions might not be interchangeable parts.
While we in no way contest the validity of OR management analytics done to date, we believe that the next steps should not be simply a continuation of existing lines of research. First, there is an underappreciation of the underlying assumptions regarding the system as applied in daily practice. Reducing the management of the operating suite (or, indeed, any complex system) to finite calculus does not adequately allow for unusual circumstances that may occur, nor does it offer instructions or heuristics to accommodate concurrent system disruptions. Second, the theories tested in the OR management literature do not necessarily translate into operational efficacy when it comes to the daily workflows in any OR, because they do not adequately address the “soft” calculations of political and human resource negotiation. OR managers are continuously challenged to assess how best to manage common and uncommon scheduling situations, overruns and underruns, block arrangements, and multisite arrangements; arrange just-in-time staffing with the appropriate skillset; effectively interleave anesthesia resources into off-site schedules; and prioritize services in a highly political environment. Although this list is not exhaustive, the astute reader should recognize now that their linear processes and decisions occur in a complex environment.
Applications of the Current Literature
The current OR management literature is based on its predictive capabilities. OR management traditionally has been broken down into three modes of thinking and decision- making processes: strategic; tactical; and operational(4):
Strategic: how we make it possible to do an operation or procedure (i.e., logistical);
Tactical: how to do a particular task (operation or procedure); and
Operational: executing the operation or procedure.
To use a boating analogy, strategic decisions are focused solely on the horizon. Here, an OR manager may be perched above on the crow’s nest, looking through a set of binoculars, with little idea of the operations below him or her. Tactical decisions center around exploiting the available tools and the environment. At the helm, the OR manager may decide how best to tack in response to the wind. Finally, the operational decisions in sailing may be construed as the mechanization of the tactical decisions (e.g., how to turn the tiller, which sails to hoist up).
In many ways, the previous focus on strategic and tactical decision has hampered the ability of an OR manager to “adapt” to the current environment. Predictive studies are important because they supply an OR manager with the constraints of the system. For instance, block allocations reduce the variability in an OR suite by specifying the service line that will operate on a specific day of the week. This tactical decision allows the agents (e.g., anesthesia healthcare team, perioperative nursing staff, surgeons, ancillary staff) to prepare for the surgical case list. However, these allocations do not inherently address operational variability on the day of surgery, such as case delays, malfunctioning equipment, or emergent add-on case. Real-world application studies on this “adaptive” component are needed.
High-Reliability Organizations: Complex Adaptive Systems
Simplistic predictive models of OR management are incomplete. They do not account for the fact that the OR is a two-tiered system dealing with stable and unstable cases. In this context, stability refers to the system, not necessarily the patient. Some processes are stable because the domain is stable: the operation, the patient, and the staff are well defined and provide constraints to the system. For example, an outpatient surgery center performs a small number of cases on healthy patients with a limited number of staff. This is an example of a niche that is well suited to predictive modeling, because there are few covariates and minimal variability. Any increase in variability will decrease system stability. A very sick patient, a new staff member, or a new technique will make predictions less accurate. In this framework, boundary conditions are not only conditions such as the patients’ medical conditions, but also the functioning of equipment, the stability of the staff, and the typical cases performed in the center.
Some of the same confusion and tension that is seen between predictive and adaptive models in OR management is also found between HROs and ultra-safe organizations (USOs). Characteristics, safety barriers, and adverse event rates in HROs and USOs are different, but organizational policies often use these terms interchangeably.(15) The fundamental difference between them is the ability and culture to limit production in order to preserve the quality of work.(16) In healthcare, the terms quality and safety often are used interchangeably. In actuality, however, they are not the same. In a USO, quality equals safety, and production is governed so that safety is never put at risk. In an HRO, quality equals reliability, and HROs typically do not limit production. In this context, “reliable” means a consistent level of performance. Safety is as high as possible with the production that is mandated, but is not explicitly a rate-limiting criterion. Examples of HROs include aircraft carriers and intensive care units. The “reliable” task can be a nighttime landing on an aircraft carrier or operating on an octogenarian with a ruptured abdominal aneurysm. In either example, the task must be attempted, and production is not limited.
Additionally, many USOs and niches provide elective services (e.g., commercial flights, outpatient surgery, blood transfusion). These USOs are well suited to both limiting workflow and rescheduling elective services. In fact, this is a major method by which USOs maintain safety. They establish boundary conditions to ensure that they will not exceed their risk profile. USOs use regulations and culture to ensure production is limited. Besides limiting production, other traits of USOs include dominant rule behavior, limited complexity, delineated areas of expertise, availability of equivalent actors, and a consistent flow of patients. By contrast, HROs are characterized by unstable recruitment of patients, deference to available local expertise, broad areas of specialized knowledge, reluctance to simplify, and complex situations. Given these prima facie similarities, USOs may be better suited for predictive models of OR management than HROs.
A two-tiered model for healthcare organizations is not a new idea. Amalberti et al.(17) suggested that some aspects of medicine are more suited to being USOs, whereas others are HROs. They argued that simply adopting tools from other industries without changing the historical and cultural precedents would have limited success. OR management may need to develop a two-tiered model as well. Systems and processes should be identified as HROs or USOs. Predictive models may be the most useful for USOs or niches in complex systems. Adaptive models may prove more useful for HROs and complex systems.
This idea is borne out in the load balancing of any given operating day. The OR schedule is, in reality, two concurrent books of business: the elective and nonelective, often with a contested hierarchy of degree of nonelectiveness. Each day, in every OR across the United States, the OR manager is faced with a chess board. The chess pieces are similar. They are typical agents: patients, surgeons, anesthesiologists, nurses, ORs, and bedspace. As in the game of chess, there are fixed moves for each piece on the board. Despite the limitation in the number of moves, there are an infinite number of possible games and two very well-defined end states: well-functioning ORs and dysfunction junction. One of the main limitations of the OR management literature is a failure to recognize that the end state is path-dependent. For instance, most healthcare consultants present data from a system where a strategic, tactical, or operational decision had a positive impact. The consultant rarely presents the data from previous initiatives that had a little impact or, worse yet, a negative impact. This brings up an important concept of survivor bias—that is, paying attention only to the initiatives that “survived” certain processes and overlooking those that did not because of their lack of visibility.(18) This bias, which is a type of selection bias, colors the operational decisions made by OR managers.(19) However, every OR has taken a different path to its current operations and organizational culture, despite starting with similar agents. A possible key to better OR management is the recognition that we need to build self-regulating systems to limit the number of end states possible. Further, we need not just limit the number of end states possible, but must prune paths so that any solution is an acceptable one. Put it this way:
Q: Is it better to be at the table or on the menu?
A: I want to be the guy that writes the menu.
“the Game of Thrones”: You Win or You Die
With this framework of CASs, we believe that there are nonreductive opportunities to create effective, transparent governing structures in OR management. Conceivably, these frameworks would modulate tactical and operational decisions. The traditional mode of hospital administrators has been to adopt the best practice model or most recent management fad. These practice models often are imposed in a top-down, champion-led manner; and, many times, there is little sustained adoption of the practices. Arguing from a bottom-up approach, we believe that the complexity framework should lead to more resilient changes.
First, organizational leaders must appreciate the basis of a knowledge organization. In psychology, there is a kind of knowledge organization known as default hierarchy,(20) which has three underlying basic principles:
Knowledge can be expressed in terms of mental structures that behave very much like rules.
These rules are in competition, so that experience causes useful rules to grow stronger and unhelpful rules to grow weaker.
Plausible new rules are generated from combinations of old rules.
From this perspective, the tactical and operational decisions made by OR managers are essentially rules. However, there should be a clear distinction here. OR managers interested in creating a paradigm shift should not confuse decisions with rules. For instance, OR managers should not decide which services should have 6-, 24-, 48-, or 72-hour release times. Instead, they should make rules (e.g., a service may request a shorter release time when they maintained a block utilization greater than 85% over a period of six months). By creating rules, the OR manager creates a self-governing, self-propagating platform and removes him- or herself from the politically charged decision-making processes common in perioperative services. Using complexity theory, the OR manager can apply Wolfram’s universality classes to OR tactical allocations (Table 1).(21) Wolfram’s Class II should be familiar to any OR manager looking at an OR block schedule. The OR block schedule is completely filled and static. When a new surgeon comes to the organization, there is little opportunity to fit that surgeon into the schedule except on nights and weekends. This type of OR block schedule is stable, but dead. OR block schedules that are completely open up to the morning of surgery may resemble Wolfram’s Class III. Here, an OR manager does not know what type of cases will be scheduled on a given day. Imagine the ramifications of this schedule and the implications when it comes to deciding which equipment will be needed and the staffing, both nursing and anesthesia, required to safely accommodate the workload. Finally, Class IV represents a live system. In this OR, the OR block allocation is fluid. Operational and tactical decisions are constantly made so that new surgeons are accommodated under the current work restraints. The simplest analogy is a garden bed. In any garden bed, an individual must constantly tend to the weeds and to the plants that are overcrowded. Removing the weeds makes room for the overcrowded plants. In short, the goal of an OR manager is push a system along so that it does not become static, but also does not explode from mismanagement. In the game of thrones you win or you die. Just as culling weeds is necessary to promote healthy growth in a garden, so too are needed nonstatic rules carefully incentivizing superior performance of not just individual departments (for limiting to such actually incentivizes chiefs of service to overhire surgeons) but of the institution’s needs and goals. Like all complex systems, including human beings, these systems adapt, grow, and learn.
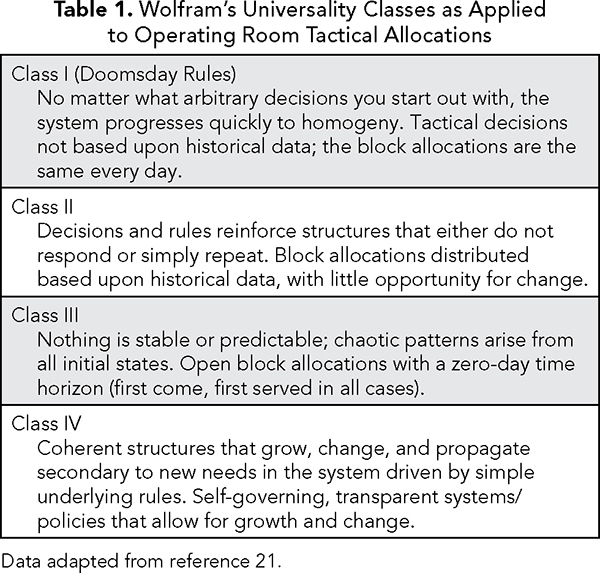
The Complex Mismatch Game
We believe that OR environments are similar to complex, living systems in that they are very close to this edge-of-chaos phase transition (Class IV). Imagine an OR manager working with a stable OR block schedule where every block has been assigned over a period of time. For a while, the block schedule works and accommodates all the agents (i.e., surgeons, nurses, and anesthesia staff). However, when a technological advancement (e.g., minimally invasive robotic surgery) or new surgical technique (e.g., transcatheter aortic valve replacement) appears, there will be perturbations in the stability of the OR block schedule. Here, one can approach policy-making on three different levels:
The conventional cost–benefit approach for each individual case;
A full institutional-political analysis, where one exhausts every potential cause and effect for the system; and
Complexity theory, wherein optimization discussion loses meaning as it puts the system in inappropriate competition with itself. The classic example is one of parents trying to optimize their behavior in terms of “us versus the kids,” which is a strange point of view if you see yourself as a family unit. One must discuss instead accommodation and coadaptation—what would be good for the unit as a whole.
Options 1 and 2, traditionally, require a strategic decision (e.g., build a new OR) or a tactical reallocation in order to integrate the robotic equipment. Similarly, newly introduced transcatheter techniques will require multiple levels of managerial decisions. Interventional cardiologists need the assistance of surgery, anesthesia, perioperative nursing, and perfusion teams in order to safely carry out their procedures. The astute OR manager should recognize that none of these decisions occur in isolation. In fact, transcatheter teams usually require additional staff and resources beyond the current budgeted allocation. Again, the traditional purview of the OR management literature rests on tactical and operational decisions. From a CAS perspective, we believe that strategic, tactical, and operational decisions will never be made in complete unison, and any decision should be understood within the context and potential contingencies of all stakeholder perspectives (Option 3).
Ultimately, the OR manager should recognize that the optimization of one decision may occur at the expense of another decision. For example, if an OR manager forgoes the decision to build a new OR to accommodate robotic equipment, that OR manager must then find time (or take away block time) from a service to “create” robotic block time. The traditional perspective that block time is stable creates little or no evolutionary pressure to change. In terms of healthcare organizations, it is as if the jobs are so subdivided that no one has any latitude; all they can do is learn how to perform one job they’ve been hired for, and nothing else. Such resistance to change by the perioperative agents permeates many large, stable organizations and is perpetuated by the current OR management literature. However, if all the agents involved in the OR participate in a process of coevolution, with each individual constantly trying to adapt to all the others, one hopes that OR “management” (or a collection of self-governing processes) continues to change and meet the ongoing and new demands of all those involved.
In today’s service-driven economies, organizations are called to cocreate value with customers(21) and business, and IT leaders are asked to deliver on this value as a part of co-leadership or digital leadership.(22,23) So, it is not unreasonable to ask stakeholders who manage ORs to address the evolving dynamics of the operating room. This can be further described by MacGregor’s Theory X and Theory Y—the models that describe two different managerial styles and their implications.(24) In Theory X organizations, decisions are made in a top-down, bureaucratic, parochial manner. The leader at the top of the organization has a vision, has vetted all the possible outcomes, and has decided on a course of action. Theory Y organizations are not necessarily led by leaders or managers, but by individuals who understand that resources, authority, and accountability need to be pushed down to the front-line workers. We believe that OR managers who view their ORs as complex adaptive systems subscribe to the Theory Y management theory.
Conclusion
The growth of the American healthcare industry has been fueled by scientific and technological advances.(25) The perioperative process has been a central component of this transformation. Before the 1990s, there was a dearth of OR management literature. In the past two decades, we have seen a proliferation of management techniques and applications of reductive analyses originally unveiled at the 1900 Paris Exposition Universelle. Today, OR managers find themselves with a legacy, or an implicit model, over 100 years old and management theories that do not necessarily apply. Snowden and Boone(26) have set forth a new leadership framework based on complexity. Their concept, the Cynefin framework, holds that leaders operate in four distinct environments: simple, complicated, complex, and chaotic. The basis of scientific management rests on the assumption that the world is ordered and predictable. In the simple and complicated environments, leaders are able to predict a cause-and-effect relationship between their actions and the consequences. However, leaders in complex environments understand that patience, a willingness to forgo a “command and control” style of management, and constant probing will eventually leads to the “emergence” of a path. The tools employed here are distinct from typical managerial techniques. Here, OR leaders open the discussion, set up self-governing limits, and create opportunities for the conditions of learning.
Recently, Ariely and Lanier(27) observed that management techniques have percolated and permeated throughout medicine. We have seen a similar rise of bureaucracy in OR management, but we believe that the reductionist, linear theories are limited in their scope and applicability. Opening the door to complexity, understanding the underpinnings of HROs, and admitting that OR systems (i.e., surgeons, nurses, anesthesia staff, and, most importantly, patients) are agents in a complex adaptive system will lead to self-governing, transparent processes that envision the OR as a living, growing, sustainable human endeavor.
References
Van de Ven AH, Poole MS. Explaining development and change in organizations. Academy of Management Review. 1995;20:510-540.
Steiger HJ, Steiger UR. A short review of game theory for neurosurgeons. Cent Eur Neurosurg. 2011;72(1):28-31. Epub 2010/05/19.
Chernov M, Pullockaran J, Vick A, Leyvi G, Delphin E. Integrated practice improvement solutions—practical steps to operating room management. J Invest Surg. 2016:1-6.
Robinson ST, Kirsch JR. Lean strategies in the operating room. Anesthesiol Clin. 2015;33:713-730..
Stacey RD. 1995. The science of complexity: an alternative perspective for strategic change processes. Strategic Management Journal. 1995;16:477-495.
Burns B. Reflections: ethics and organizational change—time for a return to Lewinian values. Journal of Change Management. 2009;9:359-381.
Holland JHH. Signals and Boundaries: Building Blocks for Complex Adaptive Systems. Cambridge, MA: MIT Press; 2014.
Flieger SP. Implementing the patient-centered medical home in complex adaptive systems: becoming a relationship-centered patient-centered medical home. Health Care Manage Rev. 2016. Epub 2016/03/05.
Kwamie A. Balancing management and leadership in complex health systems. Comment on Management matters: a leverage point for health systems strengthening in global health. Int J Health Policy Manag. 2015;4(12):849-51.
Dexter F, Wachtel RE, Epstein RH, Ledolter J, Todd MM. Analysis of operating room allocations to optimize scheduling of specialty rotations for anesthesia trainees. Anesth Analg. 2010;111:520-524.
Dexter F, Ledolter J. Managing risk and expected financial return from selective expansion of operating room capacity: mean-variance analysis of a hospital’s portfolio of surgeons. Anesth Analg. 2003;97(1):190-195.
Marion R. The Edge of Organization: Chaos and Complexity Theories of Formal Social Systems. Thousand Oaks, CA: Sage Publications; 1999.
Smith MP, Sandberg WS, Foss J, et al. High-throughput operating room system for joint arthroplasties durably outperforms routine processes. Anesthesiology. 2008;109(1):25-35.
Clark DM, Silvester K, Knowles S. Lean management systems: creating a culture of continuous quality improvement. J Clin Pathol. 2013;66:638-643.
Chassin MR, Loeb JM. High-reliability health care: getting there from here. Milbank Q. 2013;91:459-490.
Carroll JS, Rudolph JW. Design of high reliability organizations in health care. Qual Saf Health Care. 2006;15 Suppl 1:i4-9.
Amalberti R, Benhamou D, Auroy Y, Degos L. Adverse events in medicine: easy to count, complicated to understand, and complex to prevent. J Biomed Inform. 2011;44:390-394.
Elton EJ, Gruber MJ, Blake CR. Survivor bias and mutual fund performance. Rev Financ Stud. 1996;9:1097-1120.
Dexter F, Macario A, Ledolter J. Identification of systematic underestimation (bias) of case durations during case scheduling would not markedly reduce overutilized operating room time. J Clin Anesth. 2007;19(3):198-203.
The diagnostic hierarchy. The Psychiatry Letter. www.psychiatryletter.org/diagnostic-hierarchy.html. Accessed April 24, 2016.
Wolfram S. Cellular Automata and Complexity: Collected Papers. Boulder, CO: Westview Press; 1994:115-157.
Lusch RF, Vargo SL, Tanniru M. Service, value networks and learning. Journal of the Academy of Marketing Science. 2009;38(1):19-31.
Weiner J, Tanniru M, Khuntia J, Bobryk D, Naik M, Page KL. Digital leadership in action in a hospital through a real time dashboard system implementation and experience. Journal of Hospital Administration. 2016;5(4). www.sciedu.ca/journal/index.php/jha/article/view/9010
McGregor D. The Human Side of Enterprise. New York: McGraw-Hill; 1960:11-64.
Starr P. The Social Transformation of American Medicine: The Rise of a Sovereign Profession and the Making of a Vast Industry. New York: Basic Books; 1984.
Snowden DJ, Boone ME. A leader’s framework for decision making. Harvard Business Review. 2007;85(11):68.
Ariely D, Lanier WL. Disturbing trends in physician burnout and satisfaction with work-life balance: dealing with malady among the nation’s healers. Mayo Clin Proc. 2015;90:1593-1596.
Topics
Quality Improvement
Healthcare Process
Action Orientation
Related
Scope of Practice Laws for Nurse Practitioners and Physician Assistants: Impact on Healthcare Access and QualityCultural Differences: When Hospitals Own PracticesSeven Practice Assessments