Summary:
Diagnostic algorithms could save lots of time and money for health systems, but it could alter how medical decisions are made and put people out of work.
Such technologies could save lots of time and money for health systems, but it could alter how medical decisions are made and put people out of work.
The technology used by Facebook, Google and Amazon to turn spoken language into text, recognize faces and target advertising could help doctors combat one of the deadliest killers in American hospitals.
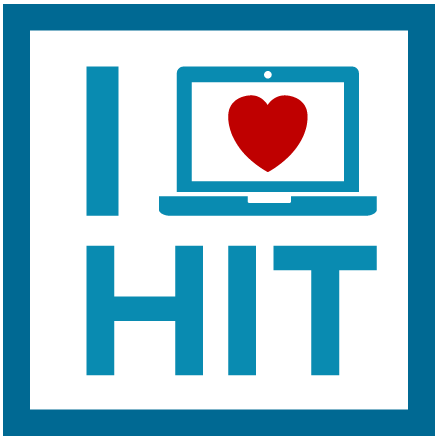
What: National Health IT Week
When: Oct. 8-12.
About: An awareness week focusing on the value of technology in health care.
Online: healthitweek.org
Clostridium difficile, a deadly bacterium spread by physical contact with objects or infected people, thrives in hospitals, causing 453,000 cases a year and 29,000 deaths in the United States, according to a 2015 study in the New England Journal of Medicine. Traditional methods such as monitoring hygiene and warning signs often fail to stop the disease.
But what if it were possible to systematically target those most vulnerable to C-diff? Erica Shenoy, MD, PhD, an infectious-disease specialist at Massachusetts General Hospital, and Jenna Wiens, a computer scientist and assistant professor of engineering at the University of Michigan, did just that when they created an algorithm to predict a patient’s risk of developing a C-diff infection, or CDI. Using patients’ vital signs and other health records, this method — still in an experimental phase — is something both researchers want to see integrated into hospital routines.
The CDI algorithm — based on a form of artificial intelligence called machine learning — is at the leading edge of a technological wave starting to hit the U.S. health care industry. After years of experimentation, machine learning’s predictive powers are well-established, and it is poised to move from labs to broad real-world applications, said Zeeshan Syed, who directs Stanford University’s Clinical Inference and Algorithms Program.
“The implications of machine learning are profound,” Syed said. “Yet it also promises to be an unpredictable, disruptive force — likely to alter the way medical decisions are made and put some people out of work.
Machine learning relies on artificial neural networks that roughly mimic the way animal brains learn.
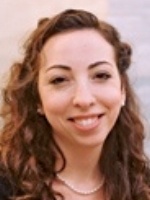
Erica Shenoy
As a fox maps new terrain, for instance, responding to smells, sights and noises, it continually adapts and refines its behavior to maximize the odds of finding its next meal. Neural networks map virtual terrains of ones and zeroes. A machine learning algorithm programmed to identify images of coffee cups might compare photos of random objects against a database of coffee cup pictures; by examining more images, it systematically learns the features to make a positive ID more quickly and accurately.
Shenoy and Wiens’ CDI algorithm analyzed a data set from 374,000 inpatient admissions to Massachusetts General Hospital and the University of Michigan Health System, seeking connections between cases of CDI and the circumstances behind them.
The records contained over 4,000 distinct variables. “We have data pertaining to everything from lab results to what bed they are in, to who is in the bed next to them and whether they are infected. We included all medications, labs and diagnoses. And we extracted this on a daily basis,” Wiens said. “You can imagine, as the patient moves around the hospital, risk evolves over time, and we wanted to capture that.”
As it repeatedly analyzes this data, the machine learning process extracts warning signs of disease that doctors may miss — constellations of symptoms, circumstances and details of medical history most likely to result in infection at any point in the hospital stay.
Such algorithms, now commonplace in internet commerce, finance and self-driving cars, are relatively untested in medicine and health care. In the U.S., the transition from written to electronic health records has been slow, and the format and quality of the data still vary by health system — and sometimes down to the medical practice level — creating obstacles for computer scientists.
RELATED: Mining Gold in Data to Boost Quality of Care, Trim Costs
But other trends are proving inexorable: Computing power has grown exponentially while getting cheaper. Once, creating a machine learning algorithm required networks of mainframe computers; now it can be done on a laptop.
Radiology and pathology will experience the changes first, experts say. Machine learning programs will most easily handle analyzing images. X-rays and MRI, PET and CT scans are, after all, masses of data. By crunching the data contained in thousands of existing scan images along with the diagnoses doctors have made from them, algorithms can distill the collective knowledge of the medical establishment in days or hours. This enables them to duplicate or surpass the accuracy of any single doctor.
Machine learning algorithms can now reliably diagnose skin cancers (from photographs) and lung cancer , and predict the risk of seizures .
Google research scientist Lily Peng, MD, PhD, led a team that developed a machine learning algorithm to diagnose a patient’s risk of diabetic retinopathy from a retinal scan. DR, a common side effect of diabetes, can lead to blindness if left untreated. The worldwide rise in diabetes rates has turned DR into a global health problem, with the number of cases expected to rise from 126.6 million in 2011 to 191 million by 2030 — an increase of nearly 51 percent. Its presence is indicated by increasingly muddy-looking scan images.
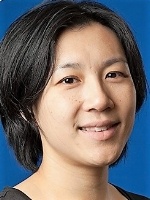
Lilly Peng
Peng’s team gathered 128,000 retinal scans from hospitals in India and the U.S. and assembled a team of 54 ophthalmologists to grade them on a five-point scale for signs of the disease. Multiple doctors reviewed each image to average out individual differences of interpretation.
Once “trained” on an initial data set with the diagnoses, the algorithm was tested on another set of data — and there it slightly exceeded the collective performance of the ophthalmologists.
Now Peng is working on applying this tool in India, where a chronic shortage of ophthalmologists means DR often goes undiagnosed and untreated until it’s too late to save a patient’s vision. (This is also a problem in the U.S., where 38 percent of adult diabetes patients do not get the recommended annual eye check for the disease, according to the Centers for Disease Control and Prevention.)
A group of Indian hospitals is testing the algorithm. Ordinarily, a scan is done, and a patient may wait days for results after a specialist — if available — reads the image. The algorithm, via software running on hospital computers, makes the results available immediately and a patient can be referred to treatment.
Last year, the Food and Drug Administration approved the first medical machine learning algorithm for commercial use by the San Francisco company Arterys . Its algorithm, “DeepVentricle,” performs in 30 seconds a task doctors typically do by hand — drawing the contours of ventricles from multiple MRI scans of the heart muscle in motion, to calculate the volume of blood passing through. That takes an average of 45 minutes. “It’s automating something that is important — and tedious,” said Carla Leibowitz, Arterys’ head of strategy and marketing.
If adopted on a broad scale, such technologies could save lots of time and money. But such change is disruptive.
“
It’s going to make a big difference in how decisions are made — things will become much more data-driven than they used to be.
John Guttag, professor of computer science at MIT
“The fact that we have identified potential ways to gut out costs is good news. The problem is the people who get gutted are not going to like it — so there will be resistance,” said Eric Topol, MD, director of the Scripps Translational Science Institute . “It undercuts how radiologists do their work. Their primary work is reading scans — what happens when they don’t have to do that?”
The shift may not put a lot of doctors out of work, said Topol, who co-authored a piece in JAMA exploring the issue. Rather, it will likely push them to find new ways to apply their expertise. They may focus on more challenging diagnoses where algorithms continue to fall short, for instance, or interact more with patients.
Beyond this frontier, algorithms can provide a more precise prognosis for the course of a disease — potentially reshaping treatment of progressive ailments or addressing the uncertainties in end-of-life care. They can anticipate fast-moving infections like CDI and chronic ailments such as heart failure.
As the U.S. population ages, heart failure will be a rising burden on the health system and on families.
“It’s the most expensive single disease as a category because of the extreme disability it causes and the high demand for care it imposes, if not managed really tightly,” said Walter “Buzz” Stewart, PhD, MPH, vice president and chief research officer at Sutter Health , a health system in Northern California. “If we could predict who was going to get it, perhaps we could begin to intervene much earlier, maybe a year or two years earlier than when it usually happens — when we admit a patient to the hospital after a cardiac event or crash.”
Stewart has collaborated on several studies aiming to address that problem. One, done with Georgia Tech computer scientist Jimeng Sun , predicts whether a patient will develop heart failure within six months, based on 12 to 18 months of outpatient medical records.
RELATED: CMO/MD/Tech Expert Bakerman on HIT’s Past, Present, Future
These tools, Stewart said, are leading to the “mass customization of health care.” Once algorithms can anticipate incipient stages of conditions like heart failure, doctors will be better able to offer treatments tailored to the patient’s circumstances.
Despite its scientific promise, machine learning in medicine remains terra incognita in many ways. It adds a new voice — the voice of the machine — to key medical decisions, for instance. Doctors and patients may be slow to accept that. Adding to potential doubts, machine learning is often a black box: Data go in, and answers come out, but it’s often unclear why certain patterns in a patient’s data point to, say, an emerging disease. Even the scientists who program neural networks often don’t understand how they reach their conclusions.
“It’s going to make a big difference in how decisions are made — things will become much more data-driven than they used to be,” said John Guttag , a professor of computer science at MIT. Doctors will rely on these increasingly complex tools to make decisions, he said, and “have no idea how they work.” And, in some cases, it will be hard to figure out why bad advice was given.
And while health data are proliferating, the quantity, quality and format vary by institution, and that affects what the algorithms “learn.”
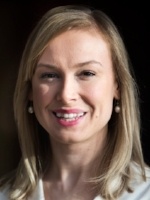
Jenna Wiens
“That is a huge issue with modeling and electronic health records,” Sun said. “Because the data are not curated for research purposes. They are collected as a byproduct of care in day-to-day operations, and utilized mainly for billing and reimbursement purposes. The data is very, very noisy.”
This also means that data may be inconsistent, even in an individual patient’s records. More important, one size does not fit all: An algorithm developed with data from one hospital or health system may not work well for another. “So you need models for different institutions, and the models become quite fragile, you might put it,” Sun said.
He is working on a National Institutes of Health grant studying how to develop algorithms that will work across institutions.
And the tide of available medical data continues to rise, tantalizing scientists. “Think about all the data we are collecting right now,” Wiens said. “Electronic health records. Hospitalizations. At outpatient centers. At home. We are starting to collect lots of data on personal monitors. These data are valuable in ways we can’t yet know.”
This article was originally published by Kaiser Health News on Feb. 14, 2018.
Topics
Technology Integration
Healthcare Process
Systems Awareness
Related
Employers Haven’t a Clue How Their Drug Benefits Are ManagedMinimizing the Potential Impact of a Crisis: PreparednessIncreasing Employee Commitment: 25 StrategiesRecommended Reading
Strategy and Innovation
Employers Haven’t a Clue How Their Drug Benefits Are Managed
Strategy and Innovation
Minimizing the Potential Impact of a Crisis: Preparedness
Operations and Policy
Increasing Employee Commitment: 25 Strategies
Operations and Policy
Staffing Shortages? Time to Consider a Virtual Assistant
Quality and Risk
Cultural Differences: When Hospitals Own Practices
Quality and Risk
Seven Practice Assessments