Abstract:
The Villages Health, a patient-centered, community-based health system automatically identified quality-improving and revenue-enhancing clinical insights buried in legacy EMR data. Using clinical natural language understanding, The Villages Health was able to identify previously hard-to-access clinical attributes for at least 15% of its patient population. The system was able to generate $2.5M in net new revenue because the clinical insights more fully captured patients’ underlying disease burdens. Finally, it was possible to employ the technology beyond disease registry creation and coding support to quality management.
This article appears ahead of publication. It will appear in the November/December 2022 issue of The Journal of Medical Practice Management.
The Villages Health, a patient-centered, community-based health system automatically identified quality-improving and revenue-enhancing clinical insights buried in legacy EMR data. Using clinical natural language understanding, The Villages Health was able to identify previously hard-to-access clinical attributes for at least 15% of its patient population. The system was able to generate $2.5M in net new revenue because the clinical insights more fully captured patients’ underlying disease burdens. Finally, it was possible to employ the technology beyond disease registry creation and coding support to quality management.
As early as 1968, Weed,(1) in his landmark article on problem-oriented medical records, identified the problem list as an important element of clinical documentation. Despite their utility, however, problem lists are notoriously incomplete.(2) Most of the clinical information within EMRs exists as unstructured information.(3) This includes free text, reports, results, notes, images, videos, and other data that are not conducive to traditional analytics. Much of this information, especially medical notes, is useful for various clinical and administrative workflows.(4) Key among these are patient summarization, quality reporting, and ensuring coding accuracy and completeness. These workflows are especially important for health systems operating in a risk-adjusted, value-based reimbursement environment. For select types of patients, perhaps most notably those with Medicare Advantage plans, quality scores and comprehensive diagnosis capture are key drivers of financial stability.(5)
A patient’s active problem list is of particular use in such pay-for-value arrangements. First, such a list helps ensure each patient is receiving the appropriate set of treatments, and also is used to measure the care against established quality metrics. Second, capitation payments or shared-savings targets typically are adjusted based on the documented and coded disease burden of the patient. Unfortunately, maintaining an accurate problem list is challenging.(6) Issues include missing diagnoses, outdated diagnoses, and duplication of diagnoses, among others.(7) Manually curating the problem list from the historical medical record is costly and carries the risk of human error. Clearly an automated system is needed.
The Villages Health (TVH) is a 75-physician multispecialty group, including 40 primary care providers, located within The Villages in central Florida. The group currently serves roughly 65,000 patients. Almost half are reimbursed under pay-for-value relationships with one of three Medicare Advantage carriers and a commercial payer. Thus, for TVH to meet its clinical and operational goals, TVH must ensure each patient’s problem list is as accurate, as comprehensive and complete, and as actionable as possible.
Objectives
The objective is to develop a cost-effective means of ensuring that each patient’s problem list reflects the complete disease burden of that patient as documented in the EMR, thus allowing for ongoing coding completeness review and gap-in-care analysis. Specific tasks include the following:
Review the medical record for significant diagnoses that currently are unreported in the patient’s problem list;
Identify test results and other findings highly suggestive of a significant diagnosis that has not yet been captured;
Locate clinical documentation in the EMR related to items listed in the problem list to allow for accuracy review; and
Present a solution to surface problem list omissions and errors to a clinical team for resolution.
Methods
The Chief Medical Officer of The Villages Health, supported by his clinical analytics and IT teams, led the project. A technology company, KAID Health (Boston, MA), provided the SaaS-based analytic solution for the project and supported the team.
The overall project focused first on identifying select conditions within the health system’s EMR that were not fully captured on the problem list or on a claim to a carrier. The selected conditions were believed to be either clinically relevant, or tied specifically to reimbursement. The initial population was all Medicare Advantage patients for whom TVH was under a fully capitated financial arrangement.
Data Source and Selection
Before the start of this project, TVH had transitioned from the eClinicalWorks EMR (West Borough, MA) to the AthenaClinicals EMR (Watertown, MA). As part of that transition, a third-party technology company, ELLKAY (Elmwood Park, NJ), archived the eClinicalWorks EMR. This archived copy of the old EMR became the initial data set on which the work was performed. The decision to use the archived eClinicalWorks EMR data versus the active Athena Health EMR was made for two reasons: 1) the eClinicalWorks EMR held the bulk of the patient data; and 2) the purpose of this investigation was to assess whether key omissions from the patient’s problem list could be identified from medical notes in a cost-effective way. It was not to test various technical means of extracting data from an active EMR. Hence the legacy data were adequate. (At the time of this writing, TVH has integrated the described solution with its Athena Heath EMR.)
The raw source data file contained both structured clinical information and unstructured medical notes. For the structured data, the team identified 7,000,000 total encounters, along with 147,000, 3,800,000, and 3,000,000 problems, medications, and lab values, respectively. After excluding duplicates and unreadable data, 113,000 problems, 2,500,000 medications, and 2,300,000 lab values were added to the database (Table 1). For the textual data, a total of 570,000 notes were identified for analysis.
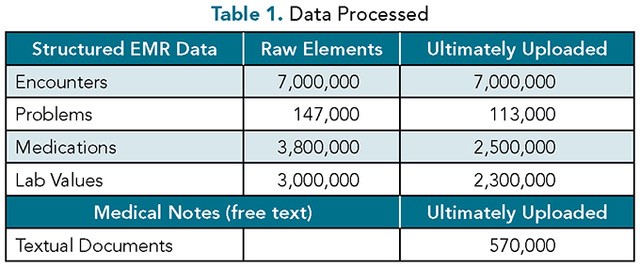
Processing the Textual Data
The 570,000 documents were analyzed utilizing the KAID Health Opportunity Identifer SaaS solution. They were processed using optical character recognition to create machine-readable text. This text was than analyzed to extract entities of clinical interest: 1) medical conditions; 2) drugs; 3) procedures and treatments; and 4) laboratory results and clinical findings. Each identified entity was then automatically assigned one or more ICD-10, CPT, RxNORM, LOINC, or SNOMED clinical codes to support subsequent analysis. The system also attempted to properly classify conditions of the patient rather than family history, “rule-outs,” or potential diagnoses (Figure 1). The resultant 250,000,000 data elements were then published in the KAID Health SaaS Environment alongside the previously uploaded structured data.
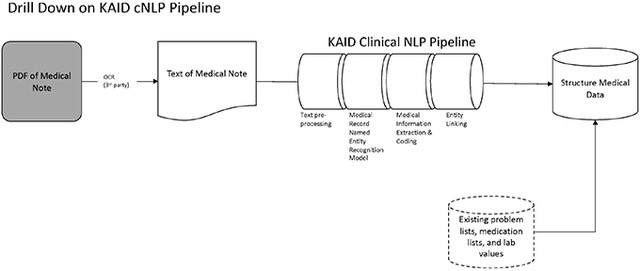
Figure 1. Overview of the KAID natural language processing pipeline, from an EMR note to structured medical data. NLP, natural language processing; OCR, Optical Character Recognition.
Analyzing the Data
The common data model populated by both the uploaded structured information and NLP-extracted insights became the basis for all subsequent analyses. The TVH team identified four medical conditions that they believed were underreported on the problem list, were clinically relevant, impacted payer reimbursement to the system, and that physicians were ready to address. This latter attribute was informed by a survey conducted of the entire medical staff by the office of the Chief Medical Officer before the start of this work. These four medical conditions were aortic atherosclerosis, neurodegenerative disease, pulmonary hypertension, and wet macular degeneration.
To find potentially omitted conditions in the data, SQL queries were formulated and run on the entire data set. Clinical staff then manually reviewed the list of suspect patients to assess whether the model accurately identified a condition noted in the medical record that was not currently on the patient’s problem list. These staff members were the same people who had, prior to this work, been the ones to manually review medical records for quality and coding purposes. When the clinician reviewers found that the AI model identified a potential new diagnosis, the patient’s primary care provider and team were engaged to review the record and make their own determination.
Results
The primary end-point was detection of relevant diagnoses in the EMR data that did not exist in the patient’s problem list. Overall, a total of 267 suspects were identified across the four initial categories (Table 2.)
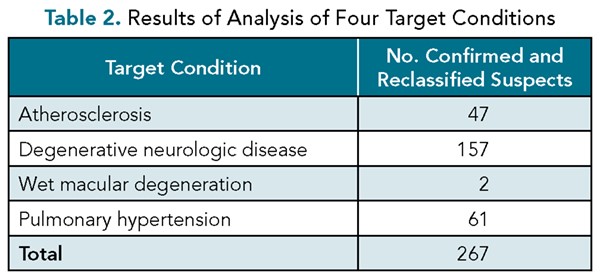
In employing natural language understanding to find these suspects, two considerations had to be balanced. The first was making sure the model identified as many diagnoses as possible that were mentioned in the patients’ charts. The second was to minimize the chance of flagging suspects who ended up not having the disease in question. Through the team’s optimization of these two requirements, the human reviewer ultimately confirmed and reclassified roughly 90% of suspects meeting initial search criteria—meaning that the positive predictive value of AI for the specified conditions was roughly 90%. This was in line with the team’s goal—that 80% to 90% of the time a suspect chart is opened, a real improvement opportunity should exist.
Just as important as model precision was the impact on clinical reviewer efficiency. The natural language understanding/natural language processing (NLU/NLP) technology, by pointing the reviewer to the potentially relevant sections of the chart, reduced this time, allowing staff to take on more work. Thus, even when a false positive occurred (10% of the time), it did not take much time to remove it from the suspect list.
Discussion
The clinical and financial implications of this work are significant. For a provider group that takes financial responsibility for the cost of care, it is imperative the medical chart be as actionable as possible. Access to well-organized EMR data has been shown to lower total cost of care and improve outcomes. Further, because the group is paid under a value-based arrangement, more complete data ensure access to appropriate and needed financial resources. Initial analysis suggests that the improved identification of diseases resulted in nearly $2,500,000 in annual net new revenue to the system. Because no new chart review labor was added to the process—those performing the chart review were already working for the practice prior to using the NLU program, the cost of the SaaS-based NLU model was the only expense incurred in obtaining this revenue increase. Thus, the health system obtained a 10-fold return on investment.
Just as important as the initial results is the infrastructure created for sustainable ongoing analysis of the medical documentation. First, although legacy data were used for this project, there is nothing inherently different about the medical text stored in the current EMR. It is the same patients treated by same physicians. Initial review suggests the gaps in the problem list were not affected by the EMR switchover. Second, although this work focused primarily on identification of issues documented, but not included, on the problem list, the same toolkit will be applied to identifying gaps in documentation versus coded problems. Finally, the ability to distill information from the medical notes is expected to streamline quality reporting efforts and support other population health and medical research aims.
This project was not the first time the provider group attempted to use natural language understanding to better summarize the charts. However, this pilot project was markedly more successful, for the following reasons:
It allowed better precision in identifying conditions of interest;
It permitted more clarity in handling unstructured data, including PDFs;
It accounted for existing provider workflows; and
It was deployed in partnership with the technology vendor, allowing rapid iteration on the approach.
Overall, the process was typical of other analytic efforts the team had previously undertaken successfully. There were, however, two sets of hurdles that had to be overcome related to this specific project—technical challenges and clinical challenges.
The first technical challenge revolved around processing the hundreds of thousands of medical notes. The sheer volume of data meant the process took roughly three weeks. The second technical challenge was developing the clinical search criteria to balance the need to identify as many target suspects as possible, while reducing the workload on the clinical team in exploring false positives. Here, the team started with easier-to-identify concepts, and then broadened them over time to more complex analyses. This approach minimized unproductive time on the part of the clinical team in reviewing suspects who ultimately proved to not have the target condition, and also built institutional momentum for the project by generating quick wins.
The clinical challenges of this project were twofold. The first was getting the project team aligned around an AI-based chart review program, including accepting the approach’s inherent opportunities and challenges. The senior leadership team introduced the concept gradually over time, managed expectations, and positioned the efforts as a chance for collective learning. The bigger clinical challenge was ensuring the resulting analytics were embraced by the line clinicians and were not burdensome on their workflows. Here again, senior clinical leadership was very methodical. To gain buy-in, senior leadership engaged all the clinicians early in the process, by conducting surveys on willingness to try new methods, providing tangible examples of the potential results, and setting achievable expectations around program adoption. To reduce workflow disruption, medical staff were leveraged to both organize the data for the clinicians and to incorporate the data into preexisting chart review processes.
The process used here is easily replicated in other clinical settings, whether the specific NLU/NLP model or another similar solution is employed. First, start with a significant clinical, financial, or operational issue faced by the organization. Examples include addressing a problematic quality measure, improving management of a specific type of patient or condition, or rectifying a systemic documentation and coding issue. The next step is to run some manual tests to determine whether better chart analytics can help. Armed with this understanding, the team should then proactively engage their staff to communicate how this approach makes everyone’s life simpler. Only then should the team scale up the analytic effort. Finally, it is worth reinforcing to the team that the goal of the project is to identify specific actions from the chart for the care team to address, rather than to just summarize the entire chart as an end in itself. This helps set appropriate expectations and keep the team focused.
The infrastructure developed for this project will extend in multiple dimensions. First, the toolkit will extract increasingly more clinical insights from the active EMR. The team already has experimented with finding several other diagnoses of interest. Second, uses of the system will expand from identifying conditions to identifying quality gaps. Finally, additional work will be done to streamline the delivery of the analytic product to frontline clinicians caring for patients. The goal at TVH is to close care gaps more efficiently while at the same time allowing the team to finish its work earlier.
References
Weed LL. Medical records that guide and teach. N Engl J Med. 1968;278(12):652-657.
Wright A, McCoy AB, Hickman TT, et al. Problem list completeness in electronic health records: a multi-site study and assessment of success factors. Int J Med Inform. 2015;84:784-790.
Kong HJ. Managing unstructured big data in healthcare system. Healthc Inform Res. 2019;25(1):1-2.
Pak HS. Unstructured data in healthcare. Healthcare Tech Outlook. 2021. https://artificialintelligence.healthcaretechoutlook.com/cxoinsights/unstructured-data-in-healthcare-nid-506.html .
Saxena S, Kaura A, Gorlin D, Kaplan J. Medicare Advantage is booming. Why are so few payers winning? BCG Global. May 23, 2019. https://www.bcg.com/publications/2019/medicare-advantage-booming-why-so-few-payers-winning .
Kanter AS. The problem with problem lists—and how EHR functionality can help fix them. Chief Healthcare Executive. May 3, 2021. www.chiefhealthcareexecutive.com/view/the-problem-with-problem-lists-and-how-ehr-functionality-can-help-fix-them .
Wang, EC-H, Wright A. Characterizing outpatient problem list completeness and duplications in the electronic health record. J Am Med Inform Assoc. 2020;27:1190-1197.
Topics
Technology Integration
Differentiation
Systems Awareness
Related
How Classical Hematology Aims to Meet the Growing Healthcare Needs of an Aging American PopulationDogs Paired With Providers at Hospitals Help Ease Staff and Patient StressHealth Policy: What’s On the Table for the Second Trump Administration?Recommended Reading
Strategy and Innovation
How Classical Hematology Aims to Meet the Growing Healthcare Needs of an Aging American Population
Strategy and Innovation
Dogs Paired With Providers at Hospitals Help Ease Staff and Patient Stress
Strategy and Innovation
Health Policy: What’s On the Table for the Second Trump Administration?
Operations and Policy
Research: How Boards Can Increase CEO Accountability
Operations and Policy
Social Loafing and the Healthcare Team
Operations and Policy
4 Questions to Ask Before You Invest In a Workplace Wellness App